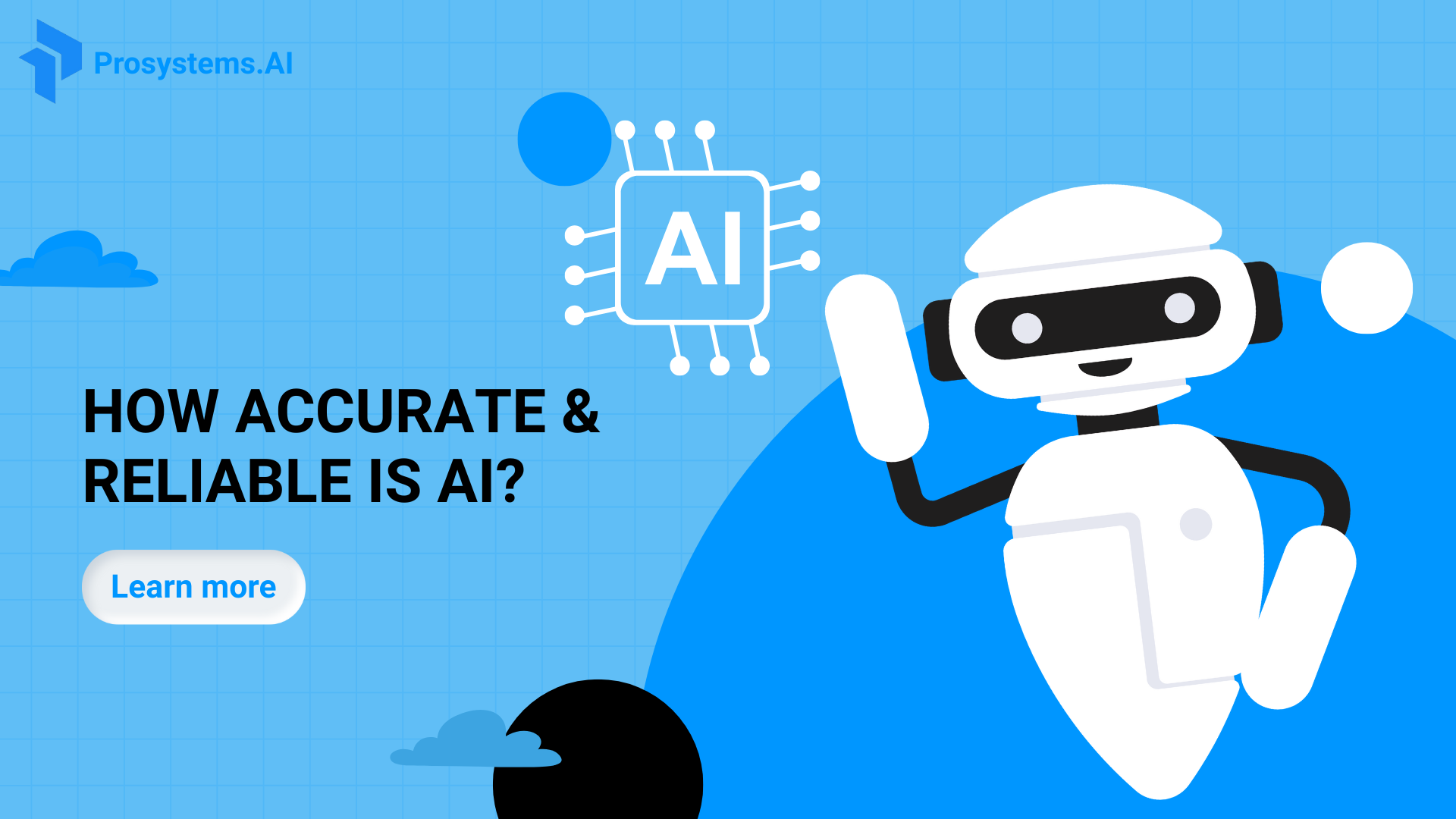
Artificial Intelligence (AI) is no longer science fiction. From chatbots and virtual assistants to facial recognition and fraud detection, AI is shaping the way we live, work, and interact with the world. But with its rise comes a critical question: how accurate and reliable is AI, really?
This article aims to unpack that question with clarity. We won’t promote a product or make bold claims. Instead, our goal is to help you understand the capabilities and limitations of AI in realistic terms.
What Do “Accuracy” and “Reliability” Mean in AI?
Before diving into real-world performance, it’s important to define two terms that often get used interchangeably:
- Accuracy refers to how often AI systems produce the correct result. For instance, does a facial recognition system identify the correct person? Does a chatbot provide the right answer?
- Reliability measures consistency. Can the AI perform well across different situations and data inputs, time after time?
A highly accurate system that is only sometimes correct isn’t reliable. And a reliable system that makes consistent mistakes isn’t accurate. In the best-case scenario, an AI system is both.
Where AI Is Highly Accurate and Reliable
AI delivers its most impressive results when applied to specific, well-defined tasks that have clear rules and are supported by large, high-quality datasets.
In these focused domains, AI systems can process information faster and, in some cases, more accurately than humans. Here are key areas where AI consistently excels:
1. Medical Imaging and Diagnostics
AI models trained on vast datasets of medical scans can detect diseases with remarkable precision.
- AI algorithms can identify early-stage cancers (such as breast cancer, lung cancer, and skin cancer) on X-rays, MRIs, and CT scans.
- Retinal disease detection: AI systems can analyze retinal images to diagnose diabetic retinopathy and age-related macular degeneration faster than traditional methods.
- In clinical studies, AI has matched or even outperformed expert radiologists in detecting bone fractures, tumors, and other abnormalities.
- These systems reduce human error and assist doctors by flagging subtle anomalies that may be overlooked.
2. Voice Recognition and Speech-to-Text
Advanced AI-powered voice assistants and transcription tools achieve near-human accuracy in understanding spoken language.
- Platforms like Google Assistant, Apple Siri, and Amazon Alexa accurately transcribe speech and understand commands, especially in quiet or structured environments.
- AI-powered transcription services (like Otter.ai and Google Meet captions) can automatically convert long conversations, lectures, and meetings into text with impressive accuracy.
- AI voice recognition systems are also tailored for different accents and dialects, improving accessibility across global user bases.
3. Email Spam Filtering
AI-driven spam filters provide a near-flawless defense against unwanted emails.
- Services like Gmail and Outlook use machine learning to identify and block spam, phishing attempts, and malware-laden emails.
- These systems analyze patterns, keywords, sender reputation, and behavior to accurately classify spam with over 99% accuracy, while minimizing false positives.
- Continuous learning allows the filters to adapt to new spam tactics in real-time.
4. Recommendation Engines
AI excels at analyzing user behavior to suggest relevant content and products.
- Platforms like Netflix, YouTube, and Spotify use AI to recommend movies, shows, videos, and music tailored to each user’s preferences.
- E-commerce sites like Amazon suggest products based on browsing history, past purchases, and even what similar users have bought.
- These recommendation systems leverage massive datasets and sophisticated algorithms to boost engagement, increase sales, and enhance user experience.
5. Industrial Automation and Robotic Process Automation (RPA)
In structured environments, AI-powered automation tools execute repetitive tasks with unparalleled consistency.
AI systems excel in environments where rules don’t change often and inputs are predictable, making them ideal for factories, warehouses, and back-office operations.
RPA software can handle routine business processes such as data entry, invoice processing, inventory management, and report generation — 24/7, without fatigue.
Manufacturing robots use AI vision systems to perform quality control, part assembly, and material handling with high precision.
Key Factors That Influence AI Accuracy
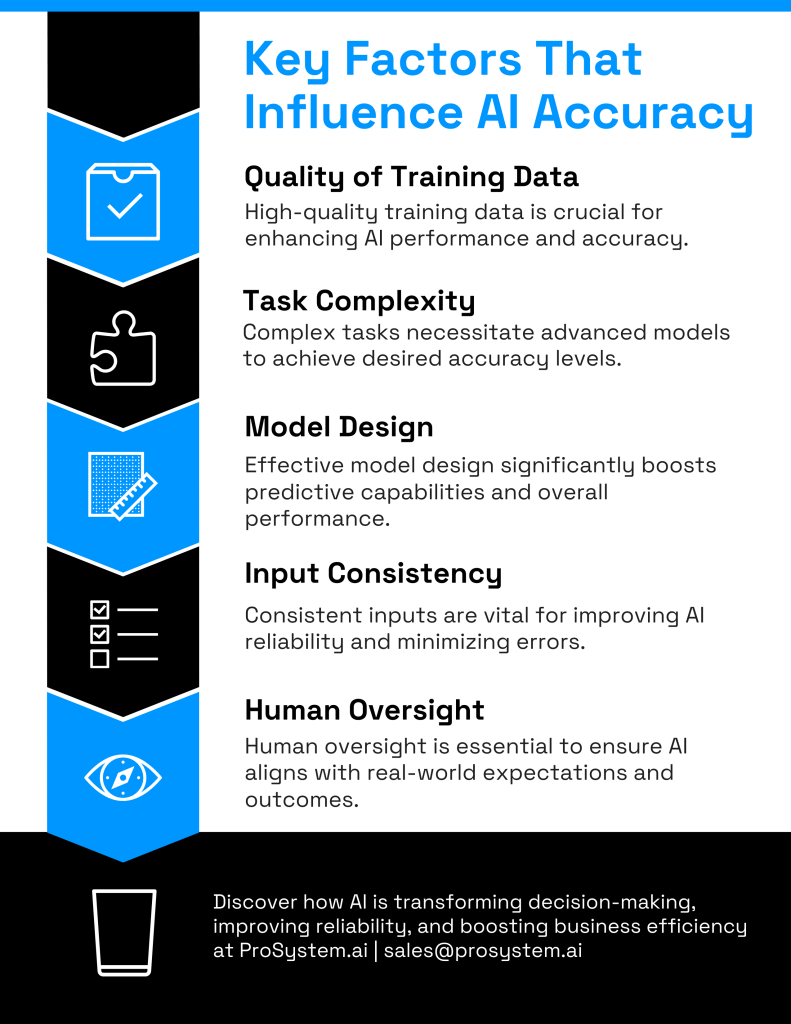
AI’s accuracy and effectiveness depend on several critical factors. When these are optimized, AI systems perform reliably and deliver value. When they’re neglected, performance suffers. Here’s a deeper look:
1. Quality of Training Data
AI systems learn patterns, relationships, and decision rules directly from data.
- If the training data is limited, the AI will struggle to generalize to new examples.
- If the data is biased, the AI will inherit and amplify those biases — leading to unfair or skewed outcomes.
- If the data contains errors, inconsistencies, or outdated information, AI predictions become inaccurate and unreliable.
- Large, diverse, and representative datasets are essential to train robust models that perform well in real-world conditions.
- Example: An AI model trained only on medical images from adults may underperform when diagnosing pediatric cases if children’s images were underrepresented in the training data.
2. Task Complexity
AI excels when tasks are simple, well-defined, and repetitive.
- Rule-based tasks (e.g., sorting emails, classifying images, processing invoices) are where AI thrives.
- High-complexity tasks requiring abstract reasoning, common sense, creativity, or emotional intelligence are still challenging for AI.
- The more ambiguity, nuance, or contextual understanding a task requires, the harder it is for AI to perform reliably.
- Example: An AI can easily label photos of cats and dogs but struggles to write nuanced poetry or provide empathetic customer service without human-like context.
3. Model Design and Algorithm Choice
Different tasks require different types of AI models — one size does not fit all.
- Deep learning models (like convolutional neural networks or transformers) excel at image recognition, natural language processing, and speech recognition.
- For structured data (e.g., spreadsheets, tables), simpler models like decision trees, random forests, or gradient boosting may outperform deep learning models — with less computational cost.
- Choosing the wrong model architecture can lead to underperformance, inefficiency, or overcomplication.
- Example: Using a deep neural network to analyze sales spreadsheets is unnecessary and inefficient compared to a simpler model optimized for tabular data.
4. Input Consistency and Environment Stability
AI performs best when it encounters consistent, familiar inputs — just like it was trained on.
- Changes in the environment can reduce accuracy, such as:
- Noisy audio (background noise or poor microphone quality)
- Slang, dialects, or typos in text-based AI systems
- New or unseen data patterns the model wasn’t exposed to during training
- The more stable and predictable the operating environment, the more reliable the AI’s performance.
- Example: A voice recognition AI trained on clean, studio-quality audio will struggle with real-world recordings full of background chatter or heavy accents unless it has been trained on diverse samples.
5. Human Oversight and Continuous Monitoring
AI systems improve and maintain reliability when humans stay involved in their lifecycle.
Example: A fraud detection AI improves when human analysts review flagged transactions, confirm false positives, and provide corrections that retrain the model.
Human-in-the-loop systems allow experts to validate, correct, and fine-tune AI predictions over time.
Regular monitoring and retraining help AI adapt to changing conditions, evolving data, and emerging patterns.
Feedback loops — where humans correct AI outputs — ensure the model remains accurate and relevant.
Key Metrics for Measuring AI Performance
Evaluating how well an AI system works isn’t as simple as checking if it’s “right or wrong.” Different applications require different types of accuracy — and each metric tells a unique story about the model’s strengths and weaknesses.
Here’s a breakdown of the most important performance metrics used to assess AI systems, with practical examples:
1. Accuracy
The overall percentage of correct predictions made by the AI system.
- Formula: (Number of correct predictions ÷ Total predictions) × 100
- When it matters: Useful when the dataset has a balanced distribution of classes.
- Example: In an image classifier that detects cats and dogs, if 950 out of 1,000 predictions are correct, the accuracy is 95%.
- Limit: Can be misleading if the dataset is imbalanced (e.g., 95% of emails are not spam — a model that predicts “not spam” every time still scores 95% accuracy, but it’s useless).
2. Precision
The percentage of positive predictions that were actually correct.
- Formula: (True Positives ÷ (True Positives + False Positives)) × 100
- When it matters: Important when false positives are costly or undesirable.
- Example: In a cancer detection system, precision measures how many patients identified as having cancer actually do — minimizing unnecessary stress and tests.
- High precision = Fewer false alarms.
3. Recall (Sensitivity or True Positive Rate)
The percentage of actual positive cases that the AI successfully identified.
- Formula: (True Positives ÷ (True Positives + False Negatives)) × 100
- When it matters: Crucial when missing a positive case is dangerous or costly.
- Example: In fraud detection, recall measures how many fraudulent transactions were caught. Missing fraud (false negatives) can be very damaging.
- High recall = Fewer missed cases.
4. F1 Score
The harmonic mean of precision and recall — balancing both metrics into a single score.
- Formula: 2 × (Precision × Recall) ÷ (Precision + Recall)
- When it matters: Ideal when you need a balance between precision and recall, and the dataset is imbalanced.
- Example: In spam filtering, you want to catch most spam (recall) and avoid blocking legitimate emails (precision). The F1 score balances both needs.
- High F1 = Good trade-off between catching positives and minimizing false alarms.
5. Error Rate
The proportion of incorrect predictions made by the AI system.
- Formula: 1 – Accuracy
- When it matters: Gives a quick sense of how often the AI is wrong.
- Example: An OCR (optical character recognition) system that misreads 50 out of 1,000 characters has an error rate of 5%.
- Low error rate = Fewer mistakes.
6. User Satisfaction (for Conversational AI and Interactive Systems)
A subjective but critical metric measuring how users perceive the AI’s usefulness and effectiveness.
- How to measure:
- Post-interaction surveys (e.g., “Was this answer helpful?”)
- Net Promoter Score (NPS)
- User engagement metrics (completion rates, session length)
- When it matters: Essential for chatbots, virtual assistants, and recommendation engines where human experience matters as much as raw accuracy.
- Example: In a customer service chatbot, even if the bot provides technically accurate answers, poor tone or confusing language can lower user satisfaction.
Why the right metric depends on the use case
Different applications prioritize different metrics, based on risks and business goals:
Use Case | Priority Metric | Why? |
---|---|---|
Fraud Detection | Recall | Catch every possible fraud, even if it means reviewing false positives |
Medical Diagnosis (Cancer Detection) | Precision + Recall (F1 Score) | Balance between catching disease and avoiding false diagnoses |
Spam Filtering | F1 Score | Balance between filtering spam and not blocking real emails |
Recommendation Engine (e-commerce) | User Satisfaction + Accuracy | Deliver relevant suggestions users actually engage with |
Autonomous Vehicles (Object Detection) | Recall | Missing an obstacle is worse than false alarms |
How Reliable Is AI Over Time?
AI systems, particularly those deployed in dynamic environments, can degrade in accuracy over time. This is due to a concept known as data drift or concept drift. As user behavior or data patterns change, an AI trained on older data becomes less effective.
To maintain reliability, AI systems must be retrained and fine-tuned regularly. This also requires ongoing monitoring, testing, and improvement cycles.
Can You Trust AI in Business Settings?
Trusting AI depends on your use case. If you’re using AI to:
- Automate simple customer service tasks (like booking or FAQs)
- Sort large volumes of structured data
- Recommend content or prioritize tasks
Then yes, AI can be very trustworthy, especially when monitored properly.
However, for tasks involving:
- Complex decision-making
- Human emotions or negotiation
- Legal or medical risk
Then AI should be used with human oversight, not as a replacement.
Frequently Asked Questions (FAQs)
1. Can AI be 100% accurate?
No. Even the best AI systems have an error margin. High-performing models in specific domains (like image recognition or medical imaging) can reach 99%+ accuracy, but achieving 100% accuracy is virtually impossible due to data imperfections and unpredictable real-world conditions.
2. Is AI reliable for customer support?
Yes — for structured, repetitive tasks like answering FAQs, booking appointments, and providing order updates. However, for complex, ambiguous, or emotionally sensitive issues, human agents remain more effective and preferred.
3. Can AI accuracy improve over time?
Yes. AI systems can improve with ongoing training, access to better and larger datasets, user feedback, and refinements to the model. Continuous learning and updates help boost both accuracy and reliability.
4. How can businesses make AI more reliable?
By regularly monitoring performance, retraining models with updated and diverse data, testing edge cases, and incorporating human review when needed. AI performs best when supervised and continuously improved over time.
5. What happens when an AI system encounters new data it hasn’t seen before?
If the new data is very different from what the AI was trained on, performance often drops. This is known as “out-of-distribution” data, and it can cause inaccurate or unexpected results. Ongoing retraining and diverse data exposure help mitigate this risk.
6. Does more data always mean higher AI accuracy?
Not necessarily. More data helps only if it is high-quality, diverse, and relevant. Feeding poor-quality, noisy, or irrelevant data into a model can actually decrease its performance. Quality beats quantity.
7. What is the role of human oversight in AI reliability?
Humans play a critical role in monitoring, validating, and fine-tuning AI outputs. Human-in-the-loop systems — where humans review and correct AI decisions — significantly improve reliability, especially in dynamic or high-risk environments.
8. How do we test AI reliability before deploying it?
AI systems are tested using a combination of validation datasets, cross-validation techniques, stress testing, and real-world pilot deployments. These methods assess how the AI performs under a variety of scenarios before going live.
9. How do companies ensure their AI systems stay accurate over time?
By implementing continuous monitoring, performing regular retraining with new and evolving data, incorporating user feedback, and updating models as business conditions or user behavior change. AI accuracy is an ongoing process, not a one-time achievement.